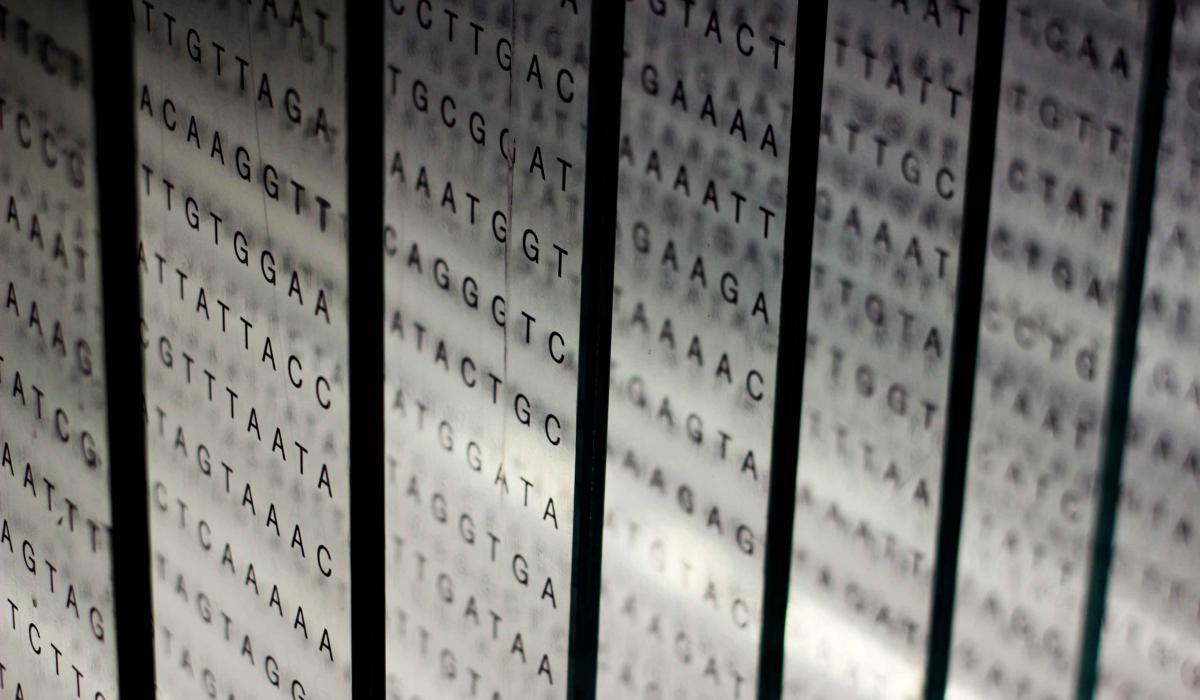
Andreas Bender
Centre for Molecular Science Informatics at the Department of Chemistry of the University of Cambridge
More and more chemical and biological information is becoming available, both in public databases as well as in company repositories.
However, how to make use of this information in chemical biology and drug discovery settings is much less clear. In this work, we will discuss how chemical and biological information from different domains – such as compound bioactivity data, pathway annotations from the bioinformatics domain, and gene expression data – can be used for a variety of purposes, such as the mode-of-action analysis from phenotypic readouts,[1,2] anticipating compound toxicities in early discovery and during lead optimization based on gene expression data[3], and for designing and selecting compounds with the desired bioactivities against a range of protein targets[4] as well as cell lines[5].
Particular attention will be paid to the integration of both chemical and biological mode-of-action data (ie those focusing on ligand-protein interactions and pathway readouts, respectively) towards understanding compound action, as well as analysing phenotypic effects from large-scale compound screens. Hence, overall, while the chemical and biological data available currently is very diverse and often not entirely understood, at least certain areas exists where we are able to show that such data can be used for understanding compound action and design which we should be able to expand upon in the future.
1. Koutsoukas A, et al. From in silico target prediction to multi-target drug design: current databases, methods and applications. J. Proteomics 2011, 74, 2554 - 2574.
2. Drakakis G, et al. Comparative mode-of-action analysis following manual and automated phenotype detection in Xenopus laevis. MedChemComm 2014, 5, 386 – 396.
3. Verbist B, et al. Using transcriptomics to guide lead optimization in drug discovery projects: Lessons learned from the QSTAR project. Drug Discov. Today 2015, 20, 505 - 513.
4. Van Westen GJP, et al. Significantly Improved HIV Inhibitor Efficacy Prediction Employing Proteochemometric Models Generated From Antivirogram Data. PLoS Comp. Biol. 2013, 9, e1002899.
5. Cortes-Ciriano, I. et al. Improved large-scale prediction of growth inhibition patterns using the NCI60 cancer cell line panel. Bioinformatics 2015, 32, 85 – 95.